Big Data is probably the latest buzzworthy term to enter into the discussions amongst technology solution providers, pundits, and enterprise information technology types, all of whom are jockeying to variously understand, explain, and offer insights as to all the fantastic opportunities, (and challenges) that Big Data presents. In case you may be late to the Big Data party, (maybe you've been goofing off too long on Pinterest to keep up), let's take a look at a basic definition of the concept from Wikipedia: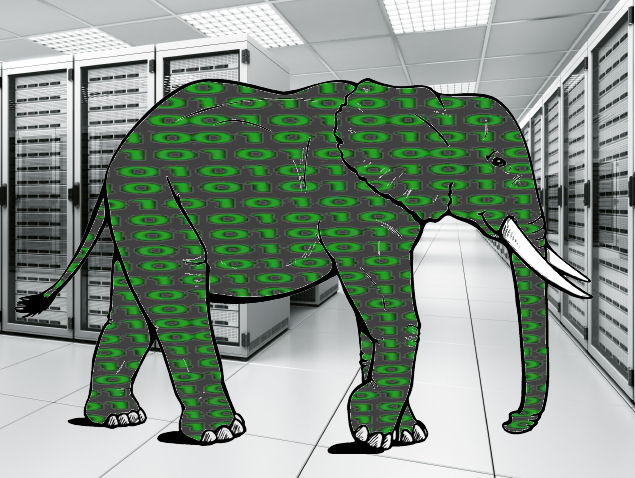
In information technology, big data consists of datasets that grow so large that they become awkward to work with using on-hand database management tools. Difficulties include capture, storage, search, sharing, analytics, and visualizing. This trend continues because of the benefits of working with larger and larger datasets allowing analysts to "spot business trends, prevent diseases, combat crime."
Scientists regularly encounter this problem in meteorology,genomics, connectomics, complex physics simulations, biological and environmental research, Internet search, finance and business informatics. Data sets also grow in size because they are increasingly being gathered by ubiquitous information-sensing mobile devices, aerial sensory technologies (remote sensing), software logs, cameras, microphones, Radio-frequency identificationreaders, and wireless sensor networks.
Got all that?
Essentially, our ability to generate and store massive amounts of data, from disparate, always-on, and almost unlimited sources, is surpassing our ability to understand, analyze, interpret, and take actions based on said data.
Where there is an identified problem with data, (massive amounts of it that don't fit traditional tools and methods of interpretation), we can expect more and better technology solutions to continue to be developed to help organizations and institutions. Doing a quick search on 'Big Data tools' already yields thousands of results, ranging from technologies and processes from some of the largest information technology companies in the world, to new ideas from start-ups trying to innovate and get a toe-hold in this emerging domain.
But like any other new technology trend, the trouble that Human Resources professionals could fall victim to is thinking that the problem of 'Big Data' is fundamentally a technical one, and that with the right or new or more powerful computing resources that suddenly 'Big Data' will start spitting out all kinds of actionable insights into their business and talent. Data has always been just that, data, and possessing more and more of it just makes it more apparent that without the ability to ask the right questions, propose the right theories, and the capability to implement the strategies suggested by all this data, then all the Big Data in the world won't mean all that much to the HR professional.
I was thinking about this after reading a recent piece titled 'Can Big Data Replace Domain Expertise?', a review of some recent articles and discussions among leading academics and data scientists debating whether or not if one possessed the data, the needed technology, and some core 'data science' skills, that actual domain experiences, (e.g. for HR or Talent data, actual experience in HR or Recruiting), would not be necessary to extract insight and actionable information from the data. In other words, "given the right data set, a data scientist with no domain expertise can out-perform experts that have been working in the field for decades."
For domain experts, this kind of a conclusion would certainly be disputed, after all, how can a techie or a statistician know more about my business, or more pointedly, my people, than I do? How can simply crunching the data take the place of the knowledge I can bring to the table?
Personally, I tend to side with the domain experts on this one, perhaps it stems from watching so many NBA games and seeing the increasing importance statistical analysis is playing in the sport and in how coaches, teams, and players are managed and evaluated. Often when I read detailed statistical analysis of a player or team that seems to be at odds with my unscientific (and likely biased) views, I often want to ask, 'But did you actually watch the games?'
But eventually the data will get to be too much, too universally known, understood, and accepted, and some of my opinions and biases might have to change if I want to continue to be seen as a relevant, or even astute judge of the NBA and its talent.
Eventually just watching the games won't be enough.
And I suspect the same thing is going to happen for managers and judges of talent inside organizations as well.