Nearing the halfway mark in 2018 and I am ready to call it right now - the topic/trend that has and will continue to dominate the HR and HR technology discussion this year is Artificial Intelligence or AI.
I will accept my share of the responsibility and blame for this no doubt. I have hit the topic numerous times on the blog, I have programmed at least seven sessions (or more) featuring AI topics for the upcoming HR Technology Conference, and the subject comes up on just about every HR Happy Hour Podcast at one point or another. In fact, one of my favorite HR Happy Hour Shows this year was the conversation I had with author and professor Joshua Gans on his new book titled Prediction Machines: The Simple Economics of Artificial Intelligence.
So if you are thinking that everyone in HR and HR tech is all in on AI you'd probably be right. And yet even with all the attention and hype, at some level I still wonder if we are talking about AI in HR enough. Or more specifically, are we talking about the important issues in AI, and are we asking the right questions before we deploy AI for HR decision making?
I thought about this again after reading an excellent piece on this very topic, titled 'Math Can't Solve Everything:Questions We Need to be Asking Before Deciding an Algorithm is the Answer' on the Electronic Frontier Foundation site. In this piece, (and you really should read it all), the authors lay out five questions that organizations should consider before turning to AI and algorithms for decision support purposes.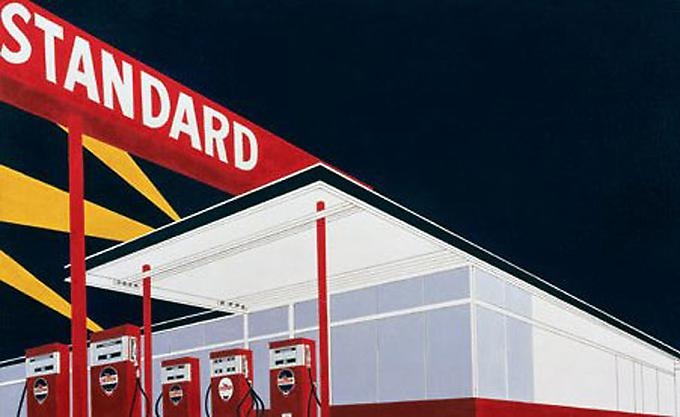
Let's take a quick look at the five questions that HR leaders should be aware of and think about, and by way of example, examine how these questions might be assessed in the context of one common 'AI in HR' use case - applying an algorithm to rank job candidates and decide which candidates to interview and consider.
1. Will this algorithm influence—or serve as the basis of—decisions with the potential to negatively impact people’s lives?
In the piece on EFF, the main example or warning cited when AI-driven processes might negatively impact people's lives is in the use of an algorithm called Compas, which has been used to predict convicted criminals likelihood to become repeat offenders. The potential danger is when the Compas score influences a judge to issue a longer prison sentence to someone the algorithm suggests is likely to repeat offend. But what if Compas is wrong? Then the convicted offender ends up spending more time than they should have in prison. So this is a huge issue in the criminal justice system.
In our HR example, the stakes are not quite so high, but they still matter. When algorithms or AI is used to rank job candidates and select candidates for interviews, those candidates who are not selected, or are rated poorly, are certainly negatively impacted by the loss of the opportunity to be considered for employment. That does not mean the AI is 'wrong' or bad necessarily, but just that HR leaders need to be open and honest that this kind of AI will certainly impact some people in a negative manner.
With that established, we can look at the remaining questions to consider when deploying AI in HR.
2. Can the available data actually lead to a good outcome?
Any algorithm relies on input data, and the 'right' input data, in order to produce accurate predictions and outcomes. In our AI in HR example, leaders deploying these technologies need to take time to assess the kinds of input data about candidates that are available and that the algorithm is considering, when determining things like rankings and recommendations. This is when we have to ask ourselves additional questions on correlation vs. causation and whether or not one data point is a genuine and valid proxy for another outcome.
In the candidate evaluation example, if the algorithm is assessing things like educational achievement or past industry experience of a candidate, are we sure that this data is indeed predictive of success for a candidate in a specific job? Again, I am not contending that we can't know which data elements are indeed predictive and valid, but that we should know them, (or at least have really strong evidence they are likely to be valid).
3. Is the algorithm fair?
At the most basic level, and the one that has the most applicability for our AI in HR example, HR leaders deploying AI have to assess whether or not the AI is fair - and the simplest way is to review if the algorithm is treating like groups similarly or disparately? Many organizations are turning to AI-powered candidate assessment and ranking processes to try to remove human bias from the hiring process and attempt to ensure fairness. HR leaders, along with their technology and provider partners have the challenge and responsibility to validate this is actually happening. 'Fairness' is a simple concept to grasp, but can be extremely hard to prove, but one that is inherently necessary in order for AI and algorithms to drive organizational and even societal outcomes. There is a lot more we can do to break this down, but for now, let's be sure we know we have, in HR, to ask this question early and often in the AI conversation.
4. How will the results (really) be used by humans?
If you deploy AI and algorithms for the purposes of ranking candidates, how will you use the AI-generated rankings? Will they be the sole determinant of which candidates get called for interviews, advance in the hiring process, and ultimately have a chance at an offer? Or will the AI rankings be just a part of the consideration and evaluation criteria for candidates, to be supplemented by 'human' review and judgement?
One of the ways the authors of the EFF piece suggest to ensure that human judgement is still a part of the process, is to engineer the algorithms in such a manner that they don't produce a single numerical value, like a candidate ranking score, but rather a narrative report and review of the candidate that a human HR person or recruiter would have to review. In that review, they would naturally apply some of their own human judgement. Bottom line, if your AI is meant to supplement humans and not replace them, you have to take active steps to ensure that is indeed the case in the organization.
5. Will people affected by these decisions have any influence over the system?
This final question is perhaps the trickiest one to answer for our AI in HR example. Job candidates who are not selected for interviews as a result of a poor or lower relative AI-driven ranking, will almost always have very little ability to influence the system or process. But rejected candidates often have valid questions as to why they were not considered for interviews and seek advice on how they could work to strengthen their skills and experiences in order to improve their chances for future opportunities. In this case, it would be important for HR leaders to have enough trust and visibility into the workings of the algorithm in order to precisely understand where any given candidate was ranked poorly. This ability to see the levers of the algorithm at work, and be able to share them in a clear and understandable manner is what HR leaders have to push their technology partners on, and be able to provide when needed.
As we continue to discuss and deploy AI in HR processes, we have to also continue to evaluate these systems and ask these and other important questions. HR decisions are big decisions. They impact people's lives in important and profound ways. They are not to be taken lightly. And if some level of these decisions are to be trusted to an algorithm, then HR leaders have to hold that algorithm (and themselves), accountable.
Have a great week!