Note: The blog is taking some well-deserved rest for the next two weeks (that is code for I am pretty much out of decent ideas, and I doubt most folks are spending their holidays reading blogs anyway), and will be re-running some of best, or at least most interesting posts from 2013. Maybe you missed these the first time around or maybe you didn't really miss them, but either way they are presented for your consideration. Thanks to everyone who stopped by in 2013!
If 2013 was the year of Robots and Automation, then the first runner up for topic of the year would probably have been Data and Analytics. The below post was my personal favorite example of the topic and what the future (the near future I bet) will hold for how data about people will be combined with data about machines and mashed up with process design in order to drive business outcomes. The piece originally ran in August 2013.
----------------------------------------------------------------------------------
Happiness and HR Data - Coming to a Delivery Truck Near You
Sometimes in all the conversation in the HR/talent space about the increased use of data, Big Data, and workforce analytics by HR leaders and organizations that practical, innovative (and possibly somewhat creepy), examples of how all this data coupled with better tools to understand it all are sometimes hard to find. Or hard to understand. Or not really specific enough that they resonate with many HR and Talent pros.
Lots of the articles and analysis about data and analytics for HR end up reading more like, 'This is going to be important', or 'This is going to be extremely important and you are not ready for it', or even 'This is going to be extremely important, you are not ready for it, but I (or my company) is ready to help you sort it out.'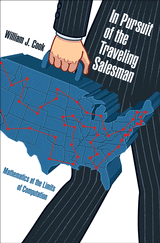
Fortunately for you, this is not one of those kind of articles.
Over the weekend I read a long-ish piece called Unhappy Truckers and Other Algorithmic Problems on the Nautilus site, that provides one of the most interesting and practical examples of how a better understanding of HR data, (among other things), is helping transportation companies plan routes, assign work, and execute managerial interventions, often before they are even needed.
At the core of most transportation and delivery problems is essentially a logistics challenge as the 'Traveling Salesman' problem. Given a fixed time period, say a day or an 8-Hour shift, and set number of destinations to visit to make sales calls, how then should the traveling salesman plan his route for the maximum efficiency.
For a salesperson making four or five stops in a day the problem is usually not that hard to solve, but for say a UPS or FedEx delivery truck driver who may have as many as 150 stops in a day - well that problem of math and logistics gets much, much more complex. And, as the piece from Nautilus describes, the Traveling Salesman problem is not only incredibly important for transportation companies to try and solve, it becomes even more complex when we factor in the the delivery drivers are actual human beings, and not just parts of an equation on a whiteboard.
Check out this excerpt from the piece to see how one (unnamed) delivery company is taking HR and workforce data, couples with the realization that indeed, people are a key element, and baking it in to the classic math problem of the Traveling Salesman:
People are also emotional, and it turns out an unhappy truck driver can be trouble. Modern routing models incorporate whether a truck driver is happy or not—something he may not know about himself. For example, one major trucking company that declined to be named does “predictive analysis” on when drivers are at greater risk of being involved in a crash. Not only does the company have information on how the truck is being driven—speeding, hard-braking events, rapid lane changes—but on the life of the driver. “We actually have built into the model a number of indicators that could be surrogates for dissatisfaction,” said one employee familiar with the program.
This could be a change in a driver’s take-home pay, a life event like a death in the family or divorce, or something as subtle as a driver whose morning start time has been suddenly changed. The analysis takes into account everything the company’s engineers can think of, and then teases out which factors seem correlated to accident risk. Drivers who appear to be at highest risk are flagged. Then there are programs in place to ensure the driver’s manager will talk to a flagged driver.
In other words, the traveling salesman problem grows considerably more complex when you actually have to think about the happiness of the salesman. And, not only do you have to know when he’s unhappy, you have to know if your model might make him unhappy. Warren Powell, director of the Castle Laboratory at Princeton University’s Department of Operations Research and Financial Engineering, has optimized transportation companies from Netjets to Burlington Northern. He recalls how, at Yellow Freight company, “we were doing things with drivers—they said, you just can’t do that.” There were union rules, there was industry practice. Tractors can be stored anywhere, humans like to go home at night. “I said we’re going to need a file with 2,000 rules. Trucks are simple; drivers are complicated."
Did you catch all the HR/talent/workforce data baked into the model described above?
Payroll, time and attendance, life events that likely would show up in the benefits admin system, scheduling are all mentioned, and I bet digging deeper into the model we'd find even more 'talent' elements like supervisor or location changes, time since a driver's last compensation increase, and maybe even 'softer' items like participation in company events or number of unread emails in their inbox.
The specifics of what bits of talent data aere being incorporated into the process matter less than the fact that in the example the HR data is being mashed up so to speak with the 'hard' data from the truck itself (which is another interesting story as well), and analyzed against past driver experiences to alert managers as to when and where an accident is more likely to occur.
There is even more to the problem than the technical observations from the truck itself, and the alogorithms' assessment of the HR/Talent data - things like Union rules and contracts factor into the equation as well.
But for me, this example of taking HR data and using it not just to try and 'predict' HR events like involuntary turnover or a better or worse performance review score, and apply it to real business outcomes, (the likelihood of accidents) represents a great example of where 'Big Data for HR' is heading.
I definitely recommend taking a few minutes this week to read the entire piece on the Nautilus site, and then think about some the next time the FedEx driver turns up with a package.